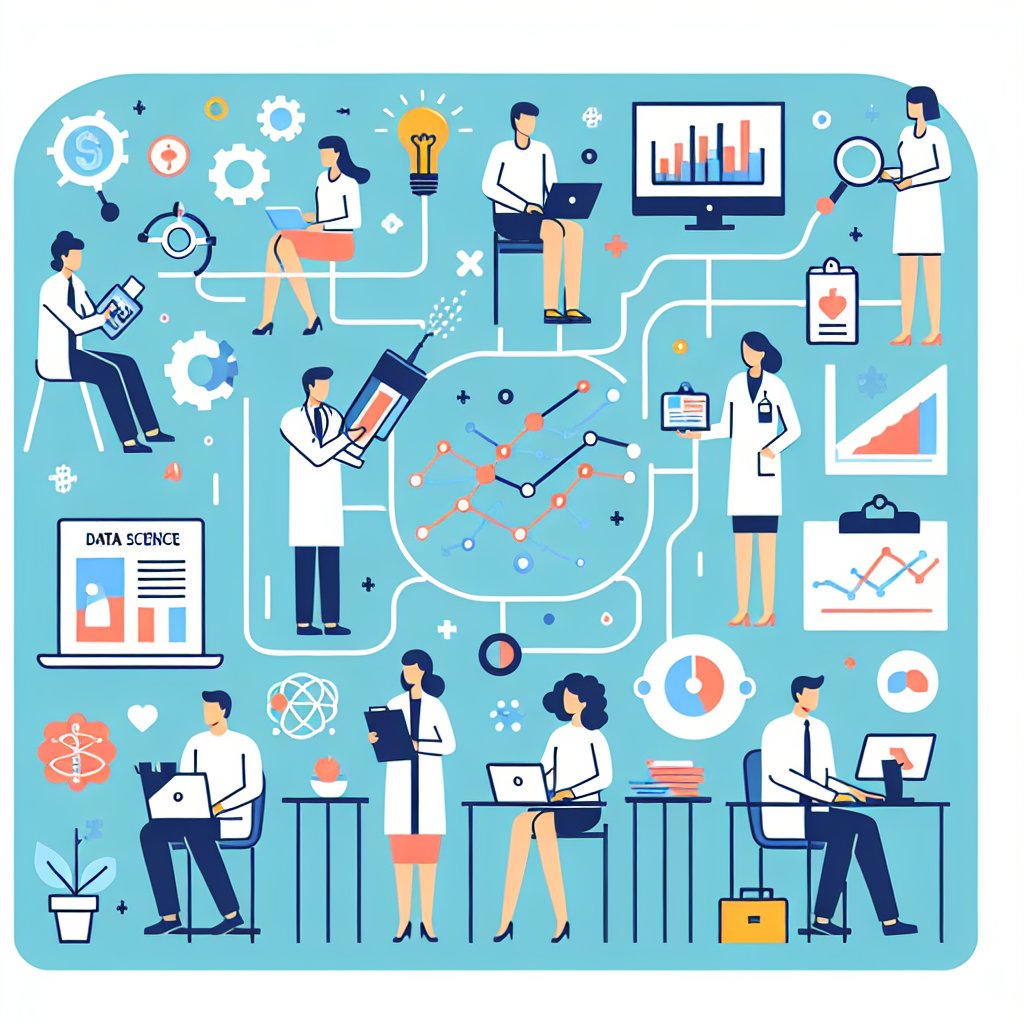
The Rise of Data Science: Transforming Industries and Shaping the Future
In today’s digital age, data has become the lifeblood of businesses across all sectors. As organizations strive to harness the power of information to gain a competitive edge, the demand for skilled professionals who can extract valuable insights from vast amounts of data has skyrocketed. Enter the data scientist – a hybrid role that combines expertise in statistics, programming, and domain knowledge to unlock the potential of big data.
What is Data Science?
Data science is an interdisciplinary field that uses scientific methods, processes, algorithms, and systems to extract knowledge and insights from structured and unstructured data. It encompasses various techniques, including machine learning, statistical analysis, and data visualization, to solve complex problems and drive informed decision-making.
The Evolution of Data Science
The concept of data science has evolved significantly over the past few decades. Here’s a brief timeline of its development:
- 1960s-1970s: Early foundations of data analysis and statistics
- 1980s-1990s: Rise of data mining and knowledge discovery in databases
- 2000s: Emergence of big data and advanced analytics
- 2010s: Explosion of machine learning and artificial intelligence
- 2020s and beyond: Integration of data science across industries and everyday life
The Growing Demand for Data Scientists
The demand for data scientists has been steadily increasing across industries, with no signs of slowing down. According to the U.S. Bureau of Labor Statistics, the employment of data scientists is projected to grow 31% from 2019 to 2029, much faster than the average for all occupations.
Factors Driving the Demand
Several factors contribute to the growing demand for data scientists:
- Exponential growth of data: With the proliferation of digital devices and technologies, the amount of data generated daily is staggering. Organizations need skilled professionals to make sense of this data deluge.
- Competitive advantage: Companies that effectively leverage data gain a significant edge over their competitors, driving the need for data-driven decision-making.
- Technological advancements: The development of powerful computing systems and sophisticated algorithms has expanded the possibilities of data analysis.
- Regulatory compliance: Increasing data privacy regulations require organizations to manage and analyze data more carefully, creating a need for expertise in data governance and ethics.
- Industry 4.0: The fourth industrial revolution, characterized by the integration of digital technologies in manufacturing and other industries, has created new opportunities for data-driven optimization.
Data Science Applications Across Industries
The versatility of data science has led to its adoption across various sectors. Let’s explore how different industries are leveraging data science to drive innovation and growth.
1. Healthcare and Life Sciences
The healthcare industry has been revolutionized by data science, with applications ranging from personalized medicine to disease prediction and prevention.
Key applications:
- Predictive diagnostics
- Drug discovery and development
- Personalized treatment plans
- Medical imaging analysis
- Hospital resource optimization
- Epidemiology and disease tracking
According to a report by Grand View Research, the global healthcare analytics market size is expected to reach $84.2 billion by 2027, growing at a CAGR of 27.5% from 2020 to 2027.
2. Finance and Banking
The financial sector has been an early adopter of data science, using it to improve risk assessment, detect fraud, and enhance customer experiences.
Key applications:
- Credit scoring and risk assessment
- Fraud detection and prevention
- Algorithmic trading
- Customer segmentation and personalization
- Anti-money laundering (AML) compliance
- Robo-advisors for wealth management
3. Retail and E-commerce
Data science has transformed the retail landscape, enabling businesses to understand customer behavior, optimize inventory, and provide personalized shopping experiences.
Key applications:
- Demand forecasting
- Price optimization
- Recommendation systems
- Supply chain optimization
- Customer churn prediction
- Sentiment analysis of customer reviews
4. Manufacturing and Industry 4.0
The manufacturing sector is leveraging data science to improve efficiency, reduce costs, and enhance product quality through predictive maintenance and process optimization.
Key applications:
- Predictive maintenance
- Quality control and defect detection
- Supply chain optimization
- Energy consumption optimization
- Product design and development
- Demand forecasting and production planning
5. Transportation and Logistics
Data science is revolutionizing the transportation industry by optimizing routes, improving safety, and enhancing the overall efficiency of logistics operations.
Key applications:
- Route optimization
- Predictive maintenance for vehicles
- Traffic flow prediction and management
- Demand forecasting for ride-sharing services
- Supply chain visibility and optimization
- Fleet management and fuel efficiency
6. Marketing and Advertising
Data science has transformed marketing strategies, enabling businesses to target customers more effectively and measure campaign performance with unprecedented accuracy.
Key applications:
- Customer segmentation and targeting
- Personalized marketing campaigns
- Attribution modeling
- Sentiment analysis of social media data
- Conversion rate optimization
- Real-time bidding for digital advertising
Essential Skills for Data Scientists
To meet the growing demand across industries, data scientists need to possess a diverse skill set that combines technical expertise with business acumen and communication skills.
Technical Skills
- Programming languages: Proficiency in languages such as Python, R, and SQL is essential for data manipulation and analysis.
- Machine learning: Understanding of various machine learning algorithms and their applications is crucial for predictive modeling and pattern recognition.
- Statistics and mathematics: A strong foundation in statistical analysis and mathematical concepts is necessary for data interpretation and model development.
- Data visualization: The ability to create compelling visualizations using tools like Tableau, PowerBI, or matplotlib is important for communicating insights effectively.
- Big data technologies: Familiarity with big data platforms like Hadoop, Spark, and cloud computing services is increasingly important for handling large-scale datasets.
Soft Skills
- Communication: The ability to explain complex technical concepts to non-technical stakeholders is crucial for driving data-driven decision-making.
- Problem-solving: Data scientists must be able to approach complex problems creatively and develop innovative solutions.
- Business acumen: Understanding the business context and aligning data science projects with organizational goals is essential for delivering value.
- Collaboration: Data scientists often work in cross-functional teams, requiring strong teamwork and interpersonal skills.
- Continuous learning: The field of data science is rapidly evolving, necessitating a commitment to ongoing learning and skill development.
Challenges in Data Science
While the opportunities in data science are vast, the field also faces several challenges that organizations and professionals must address.
1. Data Quality and Availability
One of the primary challenges in data science is ensuring the quality and availability of data. Poor data quality can lead to inaccurate insights and flawed decision-making. Organizations must invest in data governance and data management practices to ensure the reliability and accessibility of their data assets.
2. Privacy and Ethics
As data collection becomes more pervasive, concerns about privacy and ethical use of data have come to the forefront. Data scientists must navigate complex regulatory landscapes, such as GDPR and CCPA, and ensure that their practices align with ethical standards and societal expectations.
3. Talent Shortage
The rapid growth in demand for data scientists has led to a significant talent shortage. Organizations struggle to find and retain qualified professionals, leading to intense competition and rising salaries in the field.
4. Integration with Existing Systems
Implementing data science solutions often requires integration with legacy systems and processes. This can be challenging, requiring careful planning and collaboration between data scientists and IT teams.
5. Scalability and Performance
As datasets grow larger and more complex, ensuring the scalability and performance of data science solutions becomes increasingly challenging. Data scientists must optimize their algorithms and leverage distributed computing technologies to handle big data effectively.
The Future of Data Science
The field of data science is continuously evolving, driven by technological advancements and changing business needs. Here are some trends that are shaping the future of data science:
1. AutoML and No-Code Solutions
Automated Machine Learning (AutoML) and no-code platforms are making data science more accessible to non-technical users, democratizing access to advanced analytics capabilities.
2. Edge Computing and IoT
The growth of Internet of Things (IoT) devices is driving the need for edge computing and real-time analytics, creating new opportunities and challenges for data scientists.
3. Explainable AI
As AI systems become more complex, there is a growing emphasis on developing explainable AI models that can provide transparency and accountability in decision-making processes.
4. Quantum Computing
The emergence of quantum computing has the potential to revolutionize data science by solving complex optimization problems and accelerating machine learning algorithms.
5. Augmented Analytics
The integration of AI and natural language processing in analytics tools is enabling more intuitive and interactive data exploration and insights discovery.
Tools and Technologies for Data Scientists
To meet the growing demands of the field, data scientists rely on a variety of tools and technologies. Here’s an overview of some essential tools:
Programming Languages
- Python: The most popular language for data science, known for its versatility and extensive libraries.
- R: Widely used for statistical computing and graphics.
- SQL: Essential for working with relational databases.
- Julia: A high-performance language gaining popularity in scientific computing and data science.
Data Analysis and Visualization Tools
- Jupyter Notebooks: Interactive computing environment for data exploration and analysis.
- Tableau: Popular data visualization tool for creating interactive dashboards.
- Power BI: Microsoft’s business analytics tool for interactive visualizations.
- D3.js: JavaScript library for creating custom, interactive data visualizations.
Machine Learning Frameworks
- Scikit-learn: Python library for machine learning algorithms.
- TensorFlow: Open-source library for machine learning and deep learning.
- PyTorch: Deep learning framework known for its flexibility and ease of use.
- Keras: High-level neural networks API, running on top of TensorFlow.
Big Data Technologies
- Apache Hadoop: Framework for distributed storage and processing of big data.
- Apache Spark: Fast and general-purpose cluster computing system.
- Apache Kafka: Distributed streaming platform for building real-time data pipelines.
- Databricks: Unified analytics platform built on top of Apache Spark.
Cloud Platforms
- Amazon Web Services (AWS): Comprehensive cloud computing platform with various data science and machine learning services.
- Google Cloud Platform (GCP): Suite of cloud computing services, including machine learning and data analytics tools.
- Microsoft Azure: Cloud computing platform with a wide range of data science and AI services.
Career Paths in Data Science
The field of data science offers diverse career opportunities across industries. Here are some common career paths:
1. Data Analyst
Data analysts focus on collecting, processing, and analyzing data to provide insights and support decision-making. This role often serves as an entry point into the field of data science.
2. Data Scientist
Data scientists combine advanced analytical skills with domain expertise to solve complex business problems using data-driven approaches. They often work on predictive modeling and machine learning projects.
3. Machine Learning Engineer
Machine learning engineers specialize in developing and deploying machine learning models at scale. They focus on the engineering aspects of implementing machine learning solutions in production environments.
4. Data Engineer
Data engineers are responsible for designing, building, and maintaining the infrastructure and architecture for data generation, storage, and processing. They ensure that data is accessible and ready for analysis.
5. Business Intelligence Analyst
Business intelligence analysts focus on using data to drive business strategy and decision-making. They work closely with stakeholders to understand business needs and translate them into data-driven insights.
6. AI Research Scientist
AI research scientists work on advancing the field of artificial intelligence by developing new algorithms and techniques. This role often requires advanced degrees and is common in academic and research-focused organizations.
Conclusion
The growing demand for data scientists across industries reflects the increasing importance of data-driven decision-making in today’s business landscape. As organizations continue to recognize the value of extracting insights from their data assets, the role of data scientists will only become more crucial.
The field of data science offers exciting opportunities for professionals who can combine technical expertise with business acumen and communication skills. While challenges such as data quality, privacy concerns, and talent shortages persist, the ongoing technological advancements and innovative applications of data science continue to drive the field forward.
As we look to the future, the integration of data science with emerging technologies like edge computing, quantum computing, and augmented analytics promises to unlock even greater potential for innovation and value creation across industries. For those looking to enter or advance in the field of data science, continuous learning and adaptability will be key to success in this dynamic and rapidly evolving domain.
FAQs
1. What educational background is required to become a data scientist?
While there is no single path to becoming a data scientist, most professionals in the field have at least a bachelor’s degree in a quantitative field such as computer science, statistics, mathematics, or engineering. Many data scientists also hold advanced degrees (master’s or Ph.D.) in related fields. However, with the increasing availability of online courses and bootcamps, it’s also possible to enter the field through self-study and practical experience.
2. How long does it take to become a data scientist?
The time it takes to become a data scientist can vary greatly depending on your background and learning path. For someone with a relevant bachelor’s degree, it might take an additional 1-2 years of focused study and practice to develop the necessary skills. For those transitioning from other fields, it could take 2-4 years of dedicated learning and project work to build a competitive skill set.
3. What is the average salary for a data scientist?
Salaries for data scientists can vary widely based on factors such as location, industry, experience, and specific skill set. According to Glassdoor, as of 2021, the average base salary for a data scientist in the United States is around $113,000 per year. However, salaries can range from $80,000 to over $170,000, depending on the factors mentioned above.
4. What are some of the biggest challenges facing data scientists today?
Some of the major challenges include:
– Dealing with large and complex datasets
– Ensuring data quality and reliability
– Addressing ethical concerns and privacy issues
– Communicating insights effectively to non-technical stakeholders
– Keeping up with rapidly evolving technologies and methodologies
– Integrating data science solutions with existing business processes
5. How can businesses effectively incorporate data science into their operations?
To effectively incorporate data science, businesses should:
– Clearly define business objectives and align data science projects with these goals
– Invest in data infrastructure and quality management
– Foster a data-driven culture across the organization
– Build cross-functional teams that combine domain expertise with data science skills
– Start with small, high-impact projects to demonstrate value and build momentum
– Continuously evaluate and refine data science initiatives based on business outcomes
6. What is the difference between a data scientist and a data analyst?
While there can be overlap between these roles, generally:
– Data scientists typically have more advanced technical skills, particularly in areas like machine learning and statistical modeling.
– Data scientists often focus on developing predictive models and solving complex problems, while data analysts typically focus on descriptive analytics and reporting.
– Data scientists are more likely to work with unstructured data and develop custom algorithms, while data analysts often work with structured data using existing tools and methods.
7. How important is domain knowledge for a data scientist?
Domain knowledge is increasingly important for data scientists. While technical skills are crucial, understanding the business context and industry-specific challenges allows data scientists to ask the right questions, identify relevant problems, and develop solutions that deliver real business value. Many organizations now seek data scientists with expertise in their specific industry or business area.