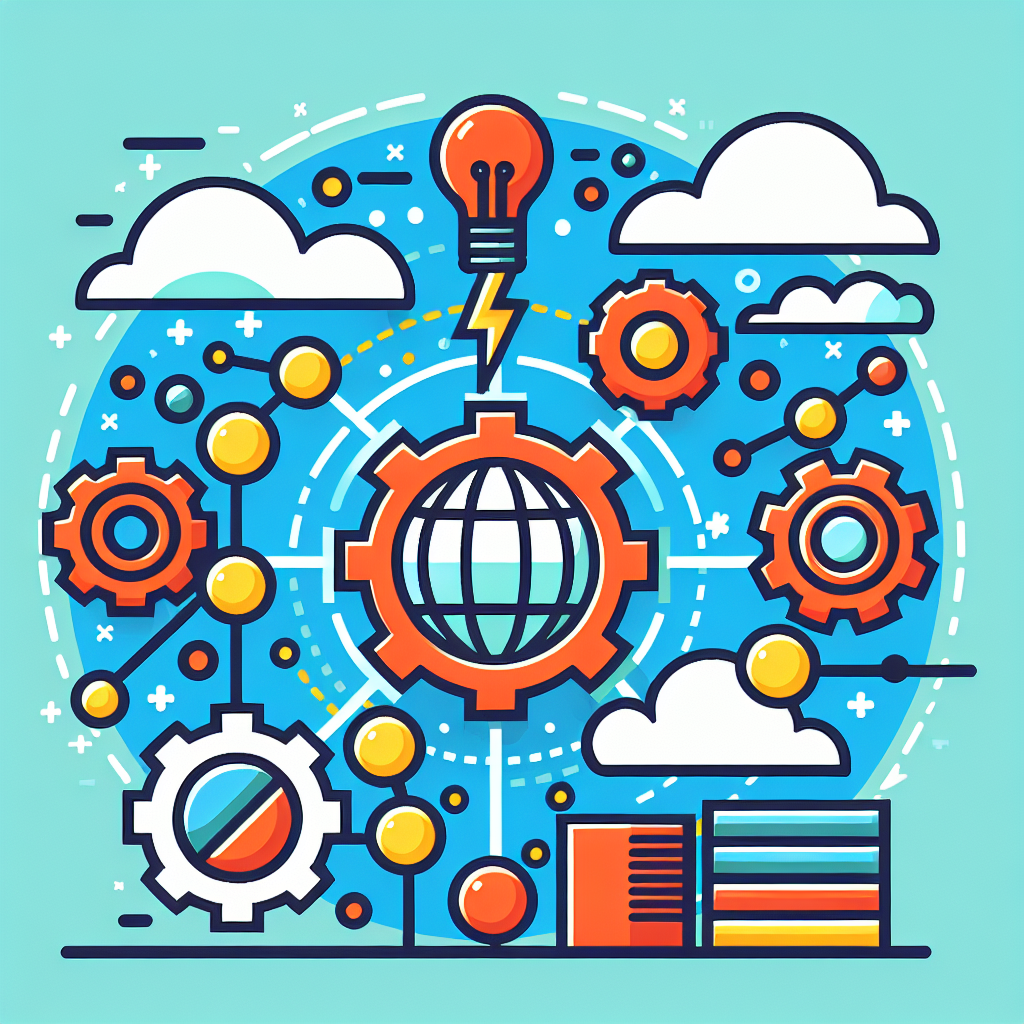
The Rise of Data Science: A Paradigm Shift in the Job Market
In an era where information is king, data science has emerged as the crown jewel of the tech industry. As we approach 2025, the impact of big data on careers is becoming increasingly apparent, reshaping the landscape of employment across various sectors. This transformation is not just limited to tech-centric roles; it’s permeating every aspect of business and society.
Understanding the Data Science Revolution
Data science combines elements of statistics, mathematics, and computer science to extract meaningful insights from vast amounts of data. With the exponential growth of digital information, the ability to analyze and interpret this data has become crucial for businesses and organizations of all sizes.
Key components of data science include:
• Machine Learning
• Artificial Intelligence
• Statistical Analysis
• Data Visualization
• Predictive Modeling
As these technologies advance, they’re creating new job opportunities and transforming existing roles across industries.
The Current State of Data Science in the Job Market
As of 2023, data science is already one of the most in-demand skill sets in the job market. According to the U.S. Bureau of Labor Statistics, the employment of data scientists is projected to grow 36% from 2021 to 2031, much faster than the average for all occupations.
Top Industries Employing Data Scientists
1. Technology
2. Finance
3. Healthcare
4. E-commerce
5. Manufacturing
The demand for data science skills is not confined to traditional tech hubs. Companies across the globe are recognizing the value of data-driven decision-making.
How Big Data Will Transform Careers by 2025
As we look towards 2025, the influence of big data on careers is set to accelerate dramatically. Here’s how various aspects of the job market will be affected:
1. Creation of New Job Roles
Big data will give rise to entirely new career paths that don’t exist today. Some potential new roles include:
• AI Ethics Officer
• Data Privacy Manager
• Quantum Machine Learning Specialist
• IoT Data Coordinator
• Augmented Analytics Consultant
These roles will require a blend of technical skills and domain expertise, highlighting the interdisciplinary nature of future careers.
2. Evolution of Existing Roles
Traditional jobs will evolve to incorporate data science elements. For example:
• Marketing Managers will become Data-Driven Marketing Strategists
• Financial Analysts will transform into Predictive Finance Modelers
• HR Professionals will evolve into People Analytics Specialists
• Sales Representatives will become AI-Assisted Sales Consultants
This evolution will require professionals to upskill and adapt to new technologies and methodologies.
3. Increased Demand for Data Literacy
By 2025, data literacy will be as fundamental as computer literacy is today. Employees across all levels will be expected to:
• Understand basic statistical concepts
• Interpret data visualizations
• Make data-driven decisions
This shift will necessitate changes in education and training programs to prepare the workforce for a data-centric future.
4. Rise of Automated Decision-Making
As AI and machine learning algorithms become more sophisticated, many routine decision-making processes will be automated. This will lead to:
• A focus on higher-level strategic thinking in management roles
• Increased importance of human creativity and emotional intelligence
• New roles centered around overseeing and auditing AI systems
5. Democratization of Data Analysis
User-friendly data analysis tools will make basic data science capabilities accessible to non-specialists. This trend will:
• Empower employees to perform their own data analysis
• Create a need for data science educators and trainers
• Shift the focus of professional data scientists to more complex problems
Industries Set for Major Disruption
While big data will impact all sectors, some industries are poised for significant transformation:
Healthcare
• Personalized medicine based on genetic data
• Predictive diagnosis using machine learning
• Optimized hospital operations through data analytics
Finance
• Algorithmic trading becoming the norm
• Fraud detection through advanced pattern recognition
• Personalized financial advice powered by AI
Retail
• Hyper-personalized shopping experiences
• Dynamic pricing strategies based on real-time data
• Predictive inventory management
Education
• Adaptive learning systems tailored to individual students
• Data-driven curriculum development
• Predictive models for student success and retention
Agriculture
• Precision farming using IoT sensors and big data
• Crop yield prediction using machine learning
• Automated farm management systems
Skills Needed to Thrive in the Data-Driven Future
To succeed in this new landscape, professionals will need to develop a range of skills:
Technical Skills
• Programming (Python, R, SQL)
• Machine Learning and AI
• Big Data Technologies (Hadoop, Spark)
• Cloud Computing
• Data Visualization
Soft Skills
• Critical Thinking
• Problem-Solving
• Communication
• Ethical Reasoning
• Adaptability
Domain Expertise
• Industry-specific knowledge
• Understanding of business processes
• Regulatory and compliance awareness
The most successful professionals will be those who can bridge the gap between technical data science skills and real-world business applications.
Preparing for the Data Science Revolution
As we approach 2025, individuals and organizations must take proactive steps to prepare for the data-driven future:
For Individuals:
1. Continuous Learning: Engage in ongoing education through online courses, bootcamps, and certifications.
2. Develop a T-shaped Skill Set: Combine depth in a specific area with breadth across related fields.
3. Build a Portfolio: Showcase your skills through personal projects and contributions to open-source initiatives.
4. Network: Connect with professionals in the data science community through conferences, meetups, and online forums.
5. Stay Informed: Keep up with the latest trends and technologies in data science and your industry.
For Organizations:
1. Invest in Training: Provide employees with opportunities to develop data science skills.
2. Foster a Data-Driven Culture: Encourage data-based decision-making at all levels of the organization.
3. Upgrade Infrastructure: Invest in the necessary technology and tools to support big data initiatives.
4. Collaborate with Academia: Partner with universities to develop curriculum and recruit talent.
5. Implement Ethical Guidelines: Develop frameworks for responsible use of AI and big data.
Challenges and Considerations
While the potential of big data is enormous, there are several challenges that need to be addressed:
Data Privacy and Security
As data becomes more valuable, ensuring its security and protecting individual privacy will be crucial. This will lead to:
• Stricter regulations like GDPR becoming global standards
• New roles focused on data governance and compliance
• Increased emphasis on ethical AI and algorithmic transparency
Bias and Fairness in AI
As AI systems become more prevalent in decision-making, addressing bias will be critical:
• Need for diverse teams in AI development
• Development of fairness-aware machine learning algorithms
• Regular audits of AI systems for bias
Bridging the Skills Gap
The rapid pace of technological change may outstrip the rate at which the workforce can adapt:
• Need for more accessible and flexible education options
• Importance of industry-academia partnerships
• Role of governments in supporting workforce transition
The Global Impact of Data Science
The data science revolution will have far-reaching effects beyond individual careers and industries:
Economic Implications
• Potential for increased productivity and economic growth
• Disruption of traditional business models
• Shift in global competitiveness based on data capabilities
Social Impact
• Potential for data-driven solutions to global challenges (climate change, healthcare, education)
• Concerns about job displacement due to automation
• Need for policies to ensure equitable distribution of benefits
Geopolitical Considerations
• Data as a strategic national asset
• Competition for data science talent on a global scale
• International cooperation and standards for data sharing and AI development
Case Studies: Data Science Success Stories
To illustrate the transformative power of data science, let’s look at some real-world examples:
1. Netflix: Personalized Content Recommendation
Netflix’s recommendation system, powered by machine learning algorithms, analyzes viewing habits to suggest content to users. This has led to:
• Increased user engagement
• Reduced churn rate
• Informed decisions on content production
2. Uber: Dynamic Pricing and Route Optimization
Uber uses big data analytics to:
• Implement surge pricing during high demand
• Optimize driver routes for efficiency
• Predict demand patterns to improve service
3. Precision Medicine Initiative
This ambitious project aims to use big data to:
• Tailor medical treatments to individual genetic profiles
• Predict disease risk based on genetic and environmental factors
• Accelerate drug discovery through data-driven research
These case studies demonstrate the wide-ranging applications of data science across different sectors and highlight the potential for innovation and improvement through data-driven approaches.
Tools and Technologies Shaping the Future of Data Science
As we look towards 2025, several emerging technologies are set to play a crucial role in the evolution of data science:
1. Quantum Computing
Quantum computers have the potential to solve complex problems exponentially faster than classical computers. This could revolutionize:
• Cryptography and cybersecurity
• Drug discovery and molecular modeling
• Financial modeling and risk assessment
2. Edge Computing
Processing data closer to its source (the “edge” of the network) will enable:
• Real-time data analysis for IoT devices
• Improved privacy and security
• Reduced latency for time-sensitive applications
3. Automated Machine Learning (AutoML)
AutoML tools automate the process of selecting and optimizing machine learning models, making data science more accessible to non-experts. This will lead to:
• Faster deployment of ML models
• Democratization of AI development
• Focus on problem-solving rather than technical implementation
4. Explainable AI (XAI)
As AI systems become more complex, there’s a growing need for transparency and interpretability. XAI aims to:
• Make AI decision-making processes understandable to humans
• Address regulatory requirements for AI transparency
• Build trust in AI systems among users and stakeholders
5. Blockchain for Data Management
Blockchain technology can enhance data management by providing:
• Immutable and transparent data records
• Decentralized data storage and sharing
• Enhanced data security and privacy
The Role of Ethics in the Data Science Revolution
As data science becomes more influential, ethical considerations will play a crucial role in shaping its development and application:
1. Ethical AI Development
• Ensuring AI systems are fair, transparent, and accountable
• Developing ethical guidelines for AI research and deployment
• Addressing issues of bias in training data and algorithms
2. Data Privacy and Consent
• Implementing robust data protection measures
• Ensuring informed consent for data collection and use
• Balancing data utility with individual privacy rights
3. Responsible Use of Predictive Analytics
• Avoiding discriminatory practices in areas like hiring and lending
• Ensuring human oversight in critical decision-making processes
• Addressing the potential for data-driven manipulation of behavior
4. Environmental Impact
• Considering the energy consumption of data centers and AI training
• Developing more energy-efficient algorithms and hardware
• Using data science to address environmental challenges
Conclusion
The data science revolution is set to reshape the career landscape dramatically by 2025. As big data becomes increasingly integral to business operations and decision-making processes, professionals across all industries will need to adapt and evolve. The demand for data science skills will extend far beyond traditional tech roles, creating new opportunities and transforming existing jobs.
Key takeaways:
1. Data literacy will become a fundamental skill across all professions.
2. New job roles will emerge, while existing ones will evolve to incorporate data science elements.
3. Industries such as healthcare, finance, and retail will see significant disruption.
4. Continuous learning and adaptability will be crucial for career success.
5. Ethical considerations will play a vital role in shaping the future of data science.
As we navigate this data-driven future, it’s essential for individuals, organizations, and educational institutions to prepare for the changes ahead. By embracing the opportunities presented by big data and developing the necessary skills, we can harness the power of data science to drive innovation, solve complex problems, and create value in ways we’ve never seen before.
The data science revolution is not just about technology; it’s about transforming how we work, make decisions, and interact with the world around us. As we approach 2025, those who can effectively leverage data science will be well-positioned to thrive in the evolving job market and contribute to shaping the future of their industries and society as a whole.
FAQs
Q1: What is data science, and why is it becoming so important?
A1: Data science is an interdisciplinary field that uses scientific methods, processes, algorithms, and systems to extract knowledge and insights from structured and unstructured data. It’s becoming increasingly important because of the exponential growth in data generation and the potential for this data to drive decision-making, innovation, and efficiency across all sectors of the economy.
Q2: Do I need to become a data scientist to benefit from the data science revolution?
A2: While specialized data science roles will be in high demand, you don’t necessarily need to become a data scientist to benefit from this revolution. Many jobs will incorporate elements of data analysis and interpretation. Developing data literacy and basic analytical skills will be valuable across various professions.
Q3: What are some good resources for learning data science skills?
A3: There are numerous online platforms offering data science courses, including Coursera, edX, and Udacity. Websites like Kaggle provide hands-on experience through data science competitions. For programming skills, resources like DataCamp and Codecademy are excellent. Additionally, many universities now offer data science degree programs and certificates.
Q4: How will data science impact jobs that aren’t traditionally tech-focused?
A4: Data science will influence non-tech jobs by introducing data-driven decision-making processes. For example, marketers will use advanced analytics for campaign optimization, HR professionals will employ predictive models for talent acquisition and retention, and healthcare providers will use data for personalized treatment plans.
Q5: What are the ethical concerns surrounding the growth of data science?
A5: Key ethical concerns include data privacy, algorithmic bias, the potential for job displacement due to automation, and the responsible use of predictive analytics. There’s also growing concern about the environmental impact of large-scale data processing and storage.
Q6: How can businesses prepare for the data science revolution?
A6: Businesses can prepare by investing in data infrastructure, providing data science training for employees, fostering a data-driven culture, and developing clear data governance policies. It’s also crucial to stay informed about emerging technologies and industry trends.
Q7: Will data science replace human decision-making?
A7: While data science will enhance and inform decision-making processes, it’s unlikely to completely replace human judgment. The most effective approach will likely be a combination of data-driven insights and human expertise, especially for complex, nuanced decisions that require contextual understanding and ethical considerations.